An avalanche of applications floods every open position. Leaders, pressed for time, turn to technology to sort through resumes. Worse still, AI tools reveal unsuspected biases.
Despite their promise of objectivity, these systems often favor male candidates. A recent study reveals that artificial intelligence models reproduce entrenched gender stereotypes. Women are thus relegated to lower-paying positions. This reality raises crucial questions about equity in recruitment.
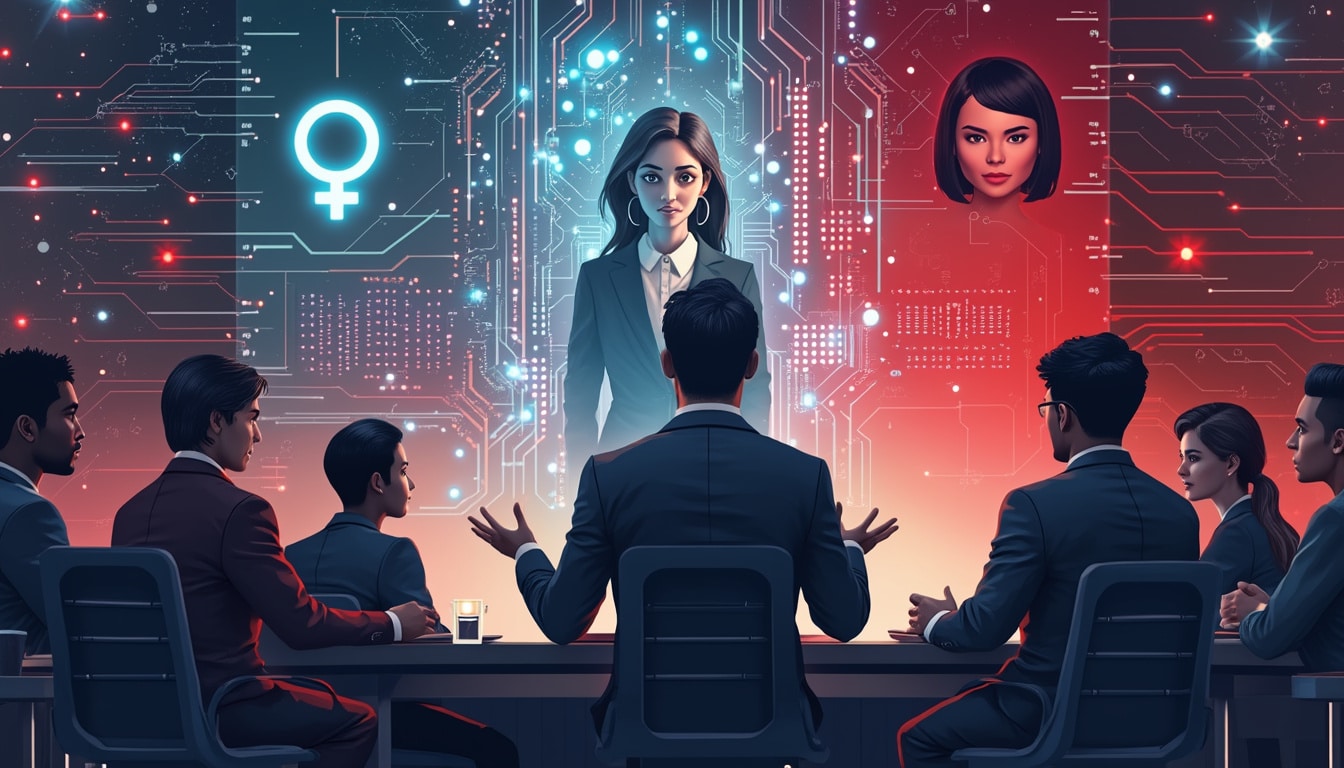
In an increasingly competitive job market, companies are turning to artificial intelligence technologies to optimize their recruitment processes. However, a recent study reveals that these tools, particularly open source hiring models, exhibit gender biases, favoring male candidates at the expense of female candidates.
Table of Contents
ToggleHow does AI influence the recruitment process?
The management of a massive flow of applications drives recruiters to adopt technological solutions to filter resumes more efficiently. According to the study conducted by Sugat Chaturvedi from the University of Ahmedabad and Rochana Chaturvedi from the University of Illinois, the open source AI tools used to screen resumes show a marked preference for male candidates. This trend is not new, but it raises crucial questions about equity and diversity in recruitment.
The researchers analyzed over 300,000 job postings in English from the national career services portal in India. By using different AI models to select between equally qualified male and female candidates, they found a clear predominance of men, particularly for better-paying positions.
What are the factors contributing to this bias?
The observed gender biases in AI models primarily stem from the training data used to develop these tools. The models learn from vast datasets collected from the web, which often reflect deeply ingrained gender stereotypes. Melody Brue from Moor Insights & Strategy highlights that these biases persist because “90 to 95% of language models are trained on datasets sourced from the web, leading to an underrepresentation of minority voices and varied professional contexts.”
Moreover, the study reveals that AI models tend to reproduce stereotypical associations between genders and professional roles, systematically leading to recommendations for women for lower-paying positions. These biased practices are not only a reflection of existing prejudices but also reinforce them, creating a vicious cycle that is hard to break.
Are there variations between different AI models?
The study tested several large language models (LLM) such as Llama-3-8B-Instruct, Qwen2.5-7BInstruct, and others. The results showed that the rates of female recommendations varied significantly depending on the model used. For instance, Llama-3.1 shows a call rate for women of 41%, which is relatively balanced, while Gemma-2-9B-Instruct reaches an impressive rate of 87.3% in favor of women, but with a higher salary penalty.
These variations indicate that not all models are uniformly biased, and some configurations may mitigate or exacerbate gender biases. Llama-3.1, for example, is also more likely to refuse to recommend a candidate when the criteria are not sufficiently clear, which can contribute to greater fairness in the selection process.
What role do personality traits play in AI model recommendations?
The study also explores the impact of personality traits on AI model recommendations. By conditioning the prompts with traits such as openness to experience, conscientiousness, extraversion, agreeableness, and emotional stability, researchers observed significant variations in refusal and recommendation rates.
For example, less agreeable models tend to reject more candidates, citing ethical concerns, while models with low conscientiousness show indifference in their choices. These results suggest that the customization of AI models can influence their decisions in complex ways, sometimes mitigating existing biases.
Case Study: Impact of Historical Personalities
To simulate more complex configurations, researchers asked the models to respond in the name of famous historical figures. Figures like Eleanor Roosevelt and Nelson Mandela helped reduce wage disparities and occupational segregation, while controversial personalities like Adolph Hitler or Joseph Stalin heightened the models’ sensitivity to gender biases.
What are the implications for businesses?
Companies adopting AI tools for recruitment must be aware of the potential biases inherent in these systems. The study underscores the importance of understanding and mitigating these biases to ensure ethical recruitment practices. Taking a proactive approach, as recommended by Melody Brue, involves creating risk assessment programs related to AI, conducting regular audits, and human intervention to balance decisions made by the algorithms.
Moreover, companies must align their practices with existing regulations, such as the EU Guidelines on Trustworthy AI, OECD recommendations on artificial intelligence, and the ethical framework and governance of AI in India. Ignoring these aspects can not only harm the company’s reputation but also lead to legal consequences.
What solutions exist to reduce gender biases in AI?
To mitigate gender biases in AI models, several strategies can be implemented. First, diversifying training data is essential. By integrating more representative data of different gender identities and eliminating stereotypes, models can learn to assess candidates based on more equitable criteria.
Second, using de-biasing techniques during the development of models can help reduce prejudices. These techniques involve adjusting algorithms to identify and correct biases before the models are deployed in real-world environments.
Finally, human supervision remains a critical component. Recruiters must be trained to recognize potential biases and intervene when AI recommendations appear unjust. Incorporating a human dimension into the recruitment process can ensure that final decisions are balanced and fair.
What are the future challenges in using AI for recruitment?
The rapid evolution of AI technologies poses constant challenges regarding regulation, ethics, and bias management. AI models are becoming increasingly sophisticated, but they inherit the imperfections of training data and algorithmic configurations. Therefore, companies need to invest in continuous technological monitoring and adapt their practices based on advancements and new scientific discoveries.
Moreover, collaboration among researchers, developers, and human resources professionals is essential to create fairer and more transparent AI solutions. By sharing best practices and working together to develop ethical standards, it is possible to build a future where AI truly contributes to better equity in recruitment.
The study highlights the persistent challenges related to gender biases in open source AI hiring models. While AI offers valuable solutions to manage the large number of applications, it is crucial to recognize and correct the biases embedded in these systems. By adopting ethical approaches and investing in more inclusive technologies, companies can ensure fairer and more diverse recruitment processes.
The issues raised by this study are all the more relevant in a context where the job market in the American tech sector shows contrasting signs. It is therefore imperative for recruiters and technology decision-makers to work together to overcome these barriers and promote true equality of opportunity.
To delve deeper into this topic and discover other analyses on strategies and challenges in recruitment and AI, check out our articles such as “Dear SaaS, what strategies have you implemented in your startup that failed to scale?” and “Monday.com positions itself as an AI-focused platform thanks to its new improvements”.
Subscribe to our newsletter to receive the latest analyses and trends in recruitment technologies and AI directly.
Certains biais #cognitifs, tels que les biais d’optimisme et de confirmation, contamineraient même nos mécanismes mentaux les plus simples, selon une étude @inserm @ENS_ULM
— Inserm (@Inserm) May 31, 2022
👉https://t.co/0BTloQKJIi pic.twitter.com/XecxYP5zfm