In a world where misinformation and disinformation proliferate, researchers are showing creativity and boldness by developing an innovative method for training large language models (LLMs) to enhance fact-checking in artificial intelligence. In the face of the growing challenges of the digital age, these advancements promise not only to increase the reliability of the information disseminated by artificial intelligences but also to strengthen public trust. With this initiative, it is a decisive step towards a future where truth and accuracy once again become the cornerstones of our interaction with technology.
Table of Contents
TogglePromising Advances in AI Evaluation
In a context where large language models (LLMs) are increasingly used, the challenges related to factual accuracy and combating bias are intensifying. Researchers are focusing on innovative training methods to overcome these persistent difficulties.
A consensus is forming around the need for adjustment of learning techniques to improve the truthfulness and reliability of information generated by artificial intelligence. One of the most recent and promising efforts lies in a method called deductive closure training (DCT).
The Stakes of Fact-Checking
On one hand, LLMs often lack factual coherence and are subject to diverse errors that can go unnoticed. Hallucinations or inaccuracies can harm many areas, including education and scientific research.
On the other hand, the need to increase the transparency and reliability of these systems has become imperative. Thus, the question of integrating high-quality data for training LLMs is clearly a major challenge.
A Revolutionary Training Method
The deductive closure training aims to enable LLMs to evaluate their own output, a process that could significantly improve fact-checking.
This process involves providing the model with basic statements from which it generates claims, some true and others false. Thus, the model is able to analyze the probability of truthfulness of these claims by charting their coherence.
The Future of Artificial Intelligence
The encouraging results of the research conducted show that the application of DCT could offer up to 26% improvement in accuracy compared to fact-checking. By integrating specific datasets and using external databases, the knowledge of these models could be significantly enhanced.
However, it remains crucial to question the ability of LLMs to become genuine fact-checking machines. Devices must evolve to avoid becoming expensive specialized tools or mere grammatical correctors.
Innovation Areas in Fact-Checking
Innovation | Impact |
Deductive closure training | Improvement of the coherence of generated data |
Use of external databases | Increase in the reliability of information |
Autonomous evaluation of accuracy | Reduction of repeated factual errors |
Selection of quality data | Optimization of LLM learning |
Increase of transparency in the process | Strengthening user trust |
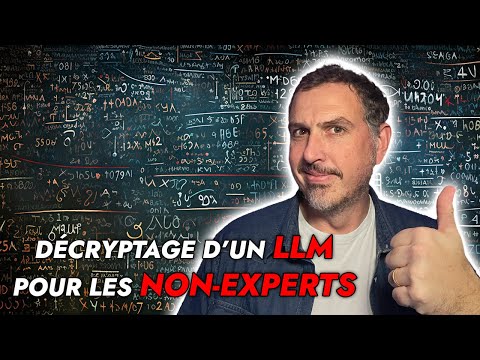
Vérification des faits | "#Factchecking : l’#IntelligenceArtificielle au service des journalistes". Interview @ioanamanol @estelle_cognacq sur @Blog_Binaire
— éduscol EMI (@eduscol_EMI) May 3, 2024
📌 Les défis à relever
📌 StatCheck, logiciel de fact checking automatiquehttps://t.co/UZhYFzddVs@Inria @SocInfoFr pic.twitter.com/Hj01tTFjPq